1. Overview
The goal of the joint COCO and Places Challenge is to study object recognition in the context of scene understanding.
Competition winners have been announced, for additional details see the workshop schedule.
2. COCO Challenges
COCO is an image dataset designed to spur object detection research with a focus on detecting objects in context. The annotations include pixel-level segmentation of object belonging to 80 categories, keypoint annotations for person instances, stuff segmentations for 91 categories, and five image captions per image. The specific tracks in the COCO 2017 Challenges are (1) object detection with bounding boxes and segmentation masks, (2) joint detection and person keypoint estimation, and (3) stuff segmentation. We describe each next.
2.1. COCO Detection Challenge
The COCO 2017 Detection Challenge is designed to push the state of the art in object detection forward. Teams are encouraged to compete in either (or both) of two object detection challenges: using bounding box output or object segmentation output. For full details of this task please see the COCO Detection Challenge page.
2.2. COCO Keypoint Challenge
The COCO 2017 Keypoint Challenge requires localization of person keypoints in challenging, uncontrolled conditions. The keypoint challenge involves simultaneously detecting people and localizing their keypoints (person locations are not given at test time). For full details of this task please see the COCO Keypoints Challenge page.
2.3. COCO Stuff Challenge
The COCO 2017 Stuff Segmentation Challenge is designed to push the state of the art in semantic segmentation of stuff classes. Whereas the COCO 2017 Detection Challenge addresses thing classes (person, car, elephant), this challenge focuses on stuff classes (grass, wall, sky). For full details of this task please see the COCO Stuff Challenge page.
3. Places Challenges
The Places Challenge will host three tracks meant to complement the COCO Challenges. The data for the 2017 Places Challenge is from the pixel-wise annotated image dataset ADE20K, in which there are 20K images for training, 2K validation images, and 3K testing images. The three specific tracks in the Places Challenge 2017 are: (1) scene parsing, (2) instance segmentation, and (3) semantic boundary detection. See the Places Challenge Page for detailed information.
4. Challenge Dates
5. Workshop Schedule - 10.29.2017
8:50 | Opening Comments | ||
---|---|---|---|
9:00 | Detection Challenge Track | Tsung-Yi Lin (Cornell Tech, Google Research) | Talk |
9:10 | Detection/Segmentation/Places Competitor | Team Megvii (Face++) | Talk |
9:30 | Detection/Segmentation Competitor | Team UCenter (CUHK & Peking University) | Talk |
9:50 | Detection/Segmentation Competitor | Team MSRA | Talk |
10:00 | Detection/Segmentation Competitor | Team FAIR | Talk |
10:10 | Morning Break: Coffee + Posters | ||
10:40 | Keypoints Challenge Track | Matteo Ronchi (Caltech) | Talk |
10:50 | Keypoints Competitor | Team Megvii (Face++) | Talk |
11:05 | Keypoints Competitor | Team OKS (Beihang University & SenseTime) | Talk |
11:20 | Stuff Challenge Track | Holger Caesar (University of Edinburgh) | Talk |
11:30 | Stuff Competitor | Team FAIR | Talk |
11:45 | Stuff Competitor | Team Oxford Active Vision Lab | —— |
12:00 | Invited Talk | Vladlen Koltun (Intel Labs) | Talk |
12:30 | Lunch | ||
14:00 | Invited Talk | Genevieve Patterson (MSR NE) | Talk |
14:15 | Invited Talk | Alexander Kirillov (University of Heidelberg & FAIR) | Talk |
14:30 | Places Challenge Track | Bolei Zhou (MIT), Hang Zhao (MIT) | Talk |
14:45 | Places Competitor | Team G-RMI (Google Research) | Talk |
15:05 | Places Competitor | Team WinterIsComing (ByteDance) | Talk |
15:25 | Places Competitor | Team CASIA_IVA_JD (Institute of Automation, Chinese Academy of Sciences, & JD) |
Talk |
15:45 | Afternoon Break: Coffee + Posters | ||
16:15 | Discussion Panel | Genevieve Patterson (MSR NE), Hang Zhao (MIT) |
6. Challenge Winners
For details on the winning entries please see the track overview slides and individual talks linked in the schedule.
1st place | 2nd place | 3rd place | 4th place | |
---|---|---|---|---|
COCO Detection: Bounding Box | Megvii | UCenter | MSRA | FAIR |
COCO Detection: Segmentation | UCenter | Megvii | FAIR | MSRA |
COCO Keypoints | Megvii | Oks | Bangbangren | — |
COCO Stuff | FAIR | G-RMI | Oxford | — |
Places Instance Segmentation | Megvii | G-RMI | BlueSky | — |
Places Scene Parsing | CASIA_IVA_JD | WinterIsComing | xdliang | — |
7. Invited Speakers

Vladlen Koltun
Intel Labs
I direct a basic research lab at Intel Labs. We are based in two locations: Santa Clara, California and Munich, Germany. We are hiring interns, postdocs, and staff researchers in both locations. We are broadly interested in visual computing and intelligent systems. Our work is usually published in computer vision, machine learning, and computer graphics conferences.
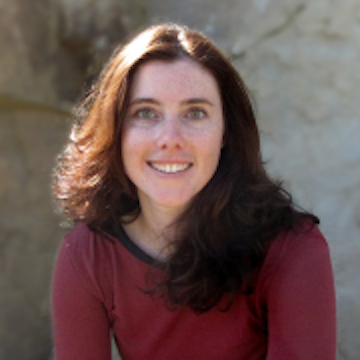
Genevieve Patterson
MSR NE
Genevieve is a postdoc at MSR New England. She studies crowd-driven Computer Vision systems and deep learning models for medical and cultural problems. Her interests include visual attribute discovery, crowd-powered dataset annotation, fine-grained object recognition, medical network interpretability, multimodal networks, and active learning. Her work has appeared at CVPR, ECCV, NIPS, IJCV, and HCOMP, where her paper introducing a system for crowdsourced fine-grained one-shot object detection was finalist for Best Paper in 2015. She received her PhD from Brown University in 2016 under the direction James Hays (now of Georgia Tech).
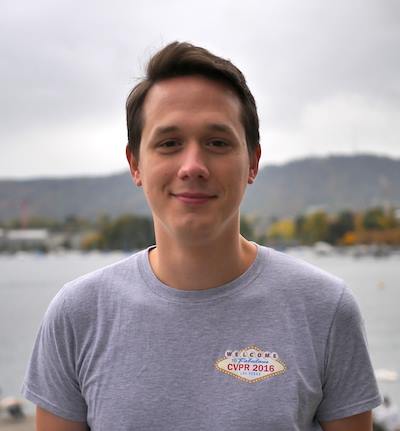
Alex Kirillov
University of Heidelberg & FAIR
Alex is a last year PhD student at the University of Heidelberg (Germany) supervised by Carsten Rother. He is currently an intern in Facebook AI Research working with Piotr Dollár, Kaiming He, and Ross Girshick. He has published numerous papers in top-tier Computer Vision and Machine Learning venues such as CVPR, ICCV, and NIPS. Alex also helped organize a CVPR tutorial on diversity in Computer Vision systems in 2016. His main research interests are deep learning models for structured output and diversity modeling for Computer Vision applications. Alex is currently looking for a full-time research position in industry starting from Spring 2018.